Data Visualisation: Crossing the line
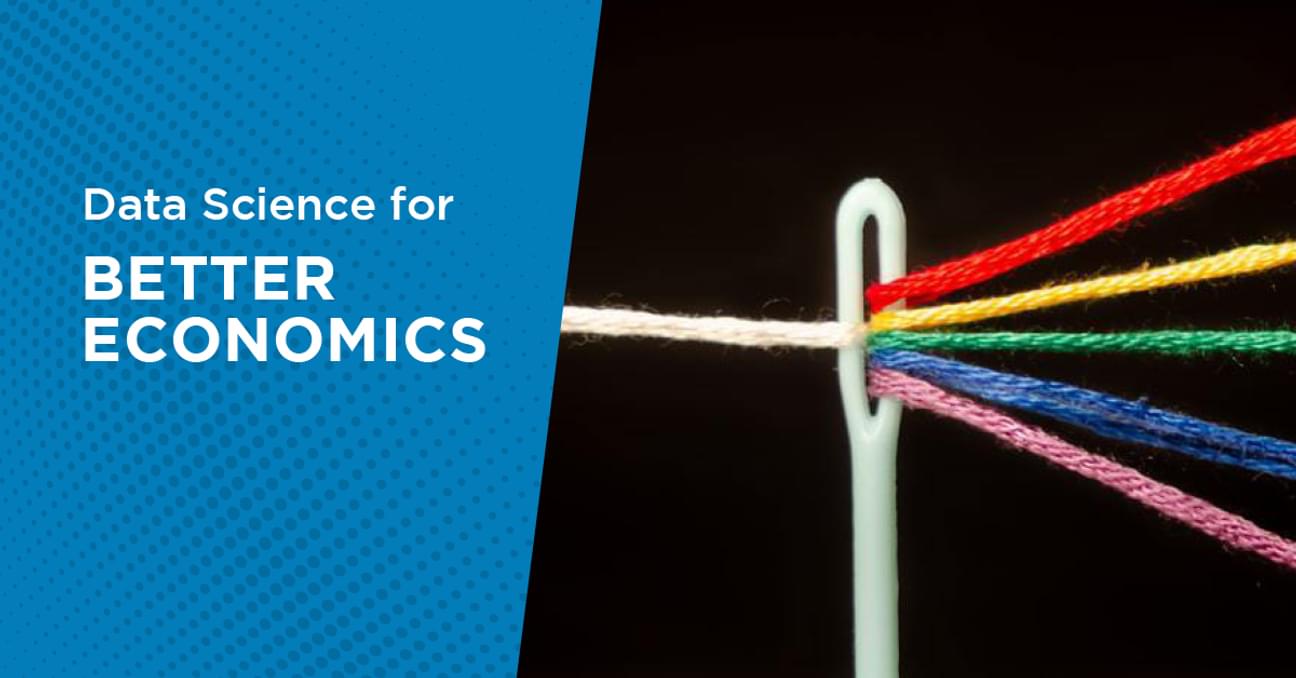
Share
Written by Tristan Salmon and James Forster
In this article, the data science team illustrate intuitive ways to explore and visualise complex data using the example of the how, where, when and who of the COVID-19 pandemic.
Traditional charts (and in particular, line charts) are very popular in economic consulting. At their best, they present a clear simple message.
But most of the situations we face aren’t simple; they’re complicated. Novel visualisation helps us explore complex data – with its myriad confounding dimensions – in clear and intuitive ways, so that we can understand different aspects of the data and identify the clear messages within it.
We use Google Mobility Data to investigate how the COVID-19 pandemic changed footfall across (i) places (such as transit, work, and home), (ii) countries and (iii) over time.
There is a lot of information to understand and convey. If we are limited to traditional approaches, we might have to scroll through slide after slide of simplistic charts, and still might fail to understand what is going on. A flexible visualisation toolbox lets us move beyond traditional approaches and explore the data in easy and intuitive ways.
We start off with a simple line chart and expand into more sophisticated visualisations to move past the former’s inherent limitations.
Traditional data visualisation
Line charts are great for some things...
The starting point for our COVID analysis is to show the evolution of footfall in transit areas (i.e. train/bus/underground stations) over time for a given place in different countries. For instance, France, Italy, Sweden and New Zealand.
Figure 1: Weekly evolution of average transit change from the baseline1
Source: Compass Lexecon analysis of Google Community Mobility Reports.
Notes:
[1] The data show how visits and length of stay at different places change compared to a baseline, using the same kind of aggregated and anonymised data used to show popular times for places in Google Maps. The baseline is defined by Google as a comparable day in the 5-week period between 3 January and Feb 6 2020. For the purposes of this article, the exact parameters of the data are not important, but you can find out more at this location.
[2] This article does not discuss the comparability of the data across countries or seasonality as the data is used for illustrative purposes only.
This line chart shows how painful things have been over the last two years for all of these four countries, and in particular, illustrates the drop due to the first lockdown in early 2020, along with Sweden’s differing strategy (immunity over lockdowns). One might think that this looks okay – and it would be if we only cared about these four countries.
... But not so great for others
Unfortunately for our chart, it isn’t enough to just look at four countries to get the full picture.
Figure 2: A few too many lines
Source: Compass Lexecon analysis of Google Community Mobility Reports.
Note: The authors were ridiculed for weeks after making this chart. They would also like to point out that this chart might be useful if we just wanted to look at the general trends or isolate the trend of a given country with respect to the distribution (for example, if all lines except the country of interest were to be greyed out, or by selecting specific lines to highlight).
With line charts, the only way to display more information (countries or places) is either to make a ridiculous number of charts or to add so many lines that it becomes illegible. As seen in the figure above, this loses its ability to convey clear insights. Given the data we want to analyse, there are three inherent limitations of using line charts.
- Limitation 1: We cannot easily add countries to the comparison without creating a mess
- Limitation 2: It is hard to investigate patterns across places
- Limitation 3: Comparing neighbouring countries is difficult
With more sophisticated visualisations, we can address these limitations.
More sophisticated data visualisation approaches
Barring all objections: Who was the worst affected?
If the purpose of the analysis is to compare countries in a given month and find the worst affected, we might show this information in a bar chart like the one below.
Static Bar charts allow us to add more countries to the analysis, but they reduce the flexibility around the number of measures that can be added (in this case, time).
Figure 3: Changes in average transit footfall in April 2020
Source: Compass Lexecon analysis of Google Community Mobility Reports.
Clearly, transit footfall in New Zealand, Italy and France was impacted the most in April 2020, whereas transit footfall in Sweden or Afghanistan was less impacted by COVID restrictions.
Whilst informative, this misses out an important dimension: evolution over time. Visualising the pandemic requires both the time and geographic dimensions to portray its impact.
Evolution over time can be shown by adding an interactive element to the bar chart and animating it such that we can show the relative rankings for each month.
Figure 4: Dynamic chart
Source: Compass Lexecon analysis of Google Community Mobility Reports.
Note: The panel on the top left of this chart can be used to cycle through the data on a monthly basis.
This interactive bar chart shows how the UK was impacted by the COVID delta variant towards the end of 2020 via its impact on public transport. For example, in August 2020, the UK was the sixth-most affected country. As the Alpha variant took hold in the later parts of the year, we can see the UK moving gradually to be the most affected country in our list by January 2021.
While this does display more countries (partly addressing Limitation 1) and how they each reacted to the pandemic over time, we still do not see how COVID impacted different places, other than just transit stations.
Turning up the heat: How did COVID affect different places across countries?
Interactive heatmaps can show the evolution over time for more countries than the simple bar charts above. Heatmaps also make it easier to switch places.
Figure 5: Heatmap
Source: Compass Lexecon analysis of Google Community Mobility Reports.
Note: The drop-down option on the top left of the chart below allows switching between visualising changes in places such as transit, work and residential (basically, how long people spent travelling, working and sitting at home wistfully looking out of the window).
This now shows how different places such as transit or retail were affected which neither the line nor bar chart allowed, at least not in a visually appealing manner. For example, people spent more time at home (“Residential”) but avoided supermarkets early in the pandemic – despite the toilet paper rush of 2020 (“Grocery and Pharmacy”).
Many of the categories show a recovery in 2021 to be closer or higher than the baseline, but one area that is still not “back to normal” is workplaces, with working from home persisting in most regions.
However, we cannot see all countries without making a giant heatmap, nor how similarly or differently neighbouring countries were affected.
Mapping out the future
How did COVID restrictions impact neighbouring countries? Which continent was most impacted at a given time?
Heatmaps are great, but for geographical data, they may make it difficult to assess the relationship between neighbouring countries (Limitation 3).
Enter, interactive maps. The map below shows the same data as in the heatmap, plus a further dimension. It allows you to see that countries that are closer to one another tend to have similar values for the various measures that we are interested in.
Figure 6: Map
Source: Compass Lexecon analysis of Google Community Mobility Reports.
Note: Technically speaking, maps like this with colours that map to values are known as chloropleths. But we just call them maps because that’s what they are.
Between March 2020 and June 2020, we see deeper reds spanning out from Italy into the rest of western Europe reflecting the decrease in mobility brought on by lockdowns and subsequent lifting of those measures showing exactly how powerful and intuitive a geographic visualisation can be.
Going beyond standard data visualisation to tell a story
We’ve discussed alternatives to traditional forms of data visualisation which provide richer information in a more intuitive manner.
Data visualisation is a rapidly evolving art form, spurred on by the increasing levels of both data availability itself and data literacy in the professional world. As economists, it is our job to represent data in as intuitive a way as possible, such that it conveys the often-imperceptible patterns in a way that makes them clear and obvious.
Visualisation is key in analysing any data and building intuition about how it fits together, which can then be complemented with econometric techniques or other predictive methods to tell the full story of the data to people with little or no prior knowledge of the data itself.
About the Data Science Team
The Compass Lexecon Data Science team was created to bring the latest developments in programming, machine learning and data analysis to economic consulting.
Sometimes this involves applying novel techniques to assess specific questions in an innovative and compelling way. For instance, running a sentiment analysis on social media content related to merging firms can be informative on their closeness of competition, and can supplement the results of a survey.
Other times it is about making work faster, more accurate, and more efficient, especially on cases which involve large datasets.
This short article is the part of a series of articles showcasing how data science can lead to more streamlined and robust economic analysis and ultimately to better decisions in competition cases. If you would like to find out more, please do not hesitate to reach out to us at datascience@compasslexecon.com.